Explanation of AI-powered multi-factor analysis used in stock selection process expending the example of love and choosing a suitable partner

Explanation of AI-powered multi-factor analysis used in stock selection process expending the example of love and choosing a suitable partner
Analytical Platform is a specialist in factor investing, the creation of multi-factor models, and the use of AI for their construction.
However, for most people, these concepts are very abstract. We will therefore try to present our concept on something close to all people, on love.
So, what would it be like if people chose their partners the way advanced analysts choose the stocks they invest in?
Establishing a Hypothesis
Hypothesize that multi-factor analysis combined with the use of machine learning will bring a higher benefit (we will be happier because we will choose partners that make us happier) than not using these tools.
The use of the mentioned tools when investing in shares brings the investor higher returns (CAGR). Thus, with the help of multifactor analysis, a person chooses factors that will earn more in the long run.
In the article, person = investor. A person chooses a partner with the best values in the factors that affect his/her happiness, just as a smart investor invests in factors that are associated with the desired price movement and looks for stocks that achieve the best values in them.
Investing in share X investing in a factor affecting the movement of the share price
How do we choose partners or stocks for the portfolio? In the first row of the table, we simply impulsively choose the better half that we just liked. The second approach is to choose a potential partner more or less at random and then tap them at least a little. In the third line, the approach begins to turn to the fact that we first choose the factors that are important to us and then look for a suitable soulmate based on them.
The approaches to investing in stocks are the same, and most investors apply those from the first two lines. First, they choose a stock and then, in the best-case scenario, find out if it could work. However, it is better to look for factors, and machine learning/AI can subsequently be used in this approach.
Partner selection | Stock selection |
We met by chance in a bar after a few drinks. The word gave word and we went for it | At FCB, we were notified that this particular stock is good, let’s go for it |
We met a potential partner by chance, but we kind of tapped him to see if it could work | We chose a stock and looked at how it stands in basic indicators (e.g. ROA, P/E, …) |
We met while doing some of our hobbies, for example in the gym. We tend to choose here rather than randomly (an important factor for us is that the potential partner is an athlete) | We bought stock from an area that we enjoy, we work in it, and we know it (an important factor for us is that the stock is from that sector) |
We used a dating app and according to some filters (factors) selected a suitable person | We set the factors and their weights (e.g., ROA with a weight of 0.5 and P/E with a weight of 0.5) and look for stocks that come out with the highest values of these ratios. |
We used an AI model based on the analysis of thousands of factors affecting stock price movements |
Technical Solution
Understanding the factors that influence our satisfaction with a partner is a fundamental building block. In the second step, we create our model that combines several of these factors. In the final step, we use AI to refine our multi-factor model.
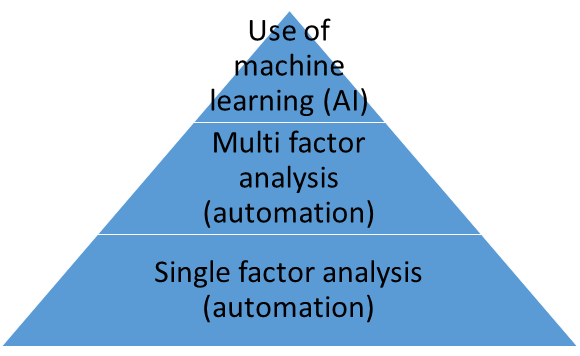
Factor Analysis
Decisions are made according to factors, there can be an infinite number of them, let’s set some.
Factors used when looking for a partner | Factors used when investing in stocks |
Look | Assets |
Hair | Asset growth |
Ass | Return of assets |
Shape of the butt | Return of assets – 1Q |
Ass size | Return of assets – 1Y |
Lifelong learning | Investment in research and development |
Generosity | Dividend payments |
There are over 10,000 known factors for making investment decisions, just as each person may be interested in something different about their partner.
Surely you’ve experienced at least in movies a rating of look from one (worst) to ten (best), this is how we would rate the results for the Look factors. Whether we prefer a smaller or bigger butt determines the direction in which the factor affects us. Let’s say we prefer bigger butts, therefore a 10 is the biggest for us. For education, for example, we might stipulate that a partner who devotes 20 hours a week to further development scores a 10. One who receives no education at all scores a zero. For stock factors, we can use the aforementioned ratios or we can create our scales for more abstract factors.
Suppose that the potential partner under consideration has the following values: Shape of the butt = 10 and Ass Size = 5.
Problem 1: In this part of the paper we encounter the first problem of using our theory in practice, since unlike a stock that we can simply buy, not every well-scored potential partner is likely to want us.
Multi-factor Analysis
A person who chooses a mate purely based on his generosity, for example, may end up being unhappy because of other qualities of the partner. Similarly, an investor who chooses a stock purely based on how fast its dividend payout increases may end up unhappy because its price increases the slowest of all.
For these reasons, more advanced investors use multi-factor analysis and try to select stocks based on multiple factors. For example, we know that what is important to us is the shape and the size of our partner’s butt, but nothing else matters.
The same works for stocks, where we can choose from several well-known models, such as the Fama-French Three-Factor Model, which selects stocks based on three factors, the size of the firm, the book value of the firm relative to its book-to-market value, and returns relative to a risk-free rate of return (e.g., U.S. bonds, excess returns on the market), the other factors are not important for this model.
It seems that picking by multifactor analysis could deliver better results in the long run than picking purely by one factor.
Assigning weights to factors
An essential task in multifactor analysis is to assign weights to the selected factors. For example, you know that butt shape and butt size are equally important to you, so you assign a value of 0.5 to each factor: Best Partner = 0.5 x Shape of the butt + 0.5 x Ass size, so the result would be 0.5 x 10 + 0.5 x 5 = 12.5.
Suppose that 12.5 is the highest value of the available partners who also accept you as their partner, and you have therefore decided to incorporate a partner in your portfolio.
Problem 2: However, the theory says that stocks with higher returns are also more volatile (experiencing more ups and downs. In the human world, this means that our counterpart might yell at us more often, making us unhappy in the short term). The solution to bring less downturns in happiness would be to have a broader portfolio, but at least in Europe, this approach is not well accepted. The investment world has an advantage in this respect and most good investment products are built on diversification.
Selection of suitable factors
For a functional multifactor model, it is important to choose the right factors that are not too influenced by each other. In the case of investing in equities, it may not be strategic to build a model using only indicators based on, for example, the book value of the company, just as it may not be strategic to select a partner based only on the shape of the butt, the size of the butt and the color of the hair, factors that are similar and have a similar effect on us. Thus, assigning an additional factor will help the robustness of our model.
Factor decay
The premise for setting the weights was that we know ourselves well and know what is important to us. Ideally, this is the case. In the worse case, we are just telling ourselves what is important to us and in practice, it is something completely different.
In this step, it is good to use automation for the first time. Let’s look at how the selected factor has affected returns from 1990 to 2019. Similarly, we can get a sense of what was important to us during this period and build a model for selecting a partner based on that.

The figure shows what an investor’s earnings would look like if he invested in the stock with the best sales per share, which measures the firm’s sales relative to the number of shares. Recall that the investor is not investing in a specific stock, but in a “factor” and is looking for the stock with the best value over time.
Problem 3: We are getting to another problematic point. Our sample investor adjusts his portfolio (stocks according to the best value of the factor in which he invests) once a month; a rebalancing horizon of several years would probably not yield very satisfactory results. In the world of relationships, however, such behavior is again not accepted, at least in our country[1], completely uncritically. Nevertheless, let us assume that the model person behaves in this way or that his partner achieves the best values for a given factor throughout our test period and that there is no reason to change it. For example, a sheik has the girl with the biggest ass in his harem, and in 30 years no other girl with a bigger ass has ever made it into the harem.
Investing in Sales per Share factor 2020 – 2023
Let us now imagine that we are choosing a partner purely based on his generosity. We know that since 1990 we have been positively influenced by the generosity of our mate. At the turn of 2019/2020, we met a new partner who was more generous than the previous one and decided to make a change. But alas, in 2020 we read in a magazine that financial independence is far better, we started earning our own money and suddenly generosity has the exact opposite effect on us, it annoys us.
And that’s exactly what happened with the impact of the Sales per Share factor on the price of a firm/company in 2020-2023. Firms with worse sales per share grew in price faster than those with better ones.

Even though we know ourselves, we cannot see into the future. The same is true for factor investing. Three years is a pretty short period in investing, and we can expect to see a return to the 1990 – 2019 performance in the years ahead. It’s hard to say how our role model will fare with perceptions of generosity.
A proper multifactor model should therefore be sufficiently robust, i.e. not to include correlated factors and to work in different market/personal conditions, i.e. in times when we are more or less financially independent, which is what machine learning helps us to do.
However, we can do without AI. The vast majority of investors still make decisions based on a few factors that they don’t even know have a real impact on stock price movements. Just by looking at how a factor has affected stock price movements over a long time series, and somehow using that information in our investment decisions, we are doing better than most investment companies.
Using automation, we can then select the factors that have influenced price movements at a given time and invest in them, i.e. create, for example, a robust strategy from 20 factors that have historically significantly influenced price movements. If we want to take our investing to the next level, it is advisable to familiarize ourselves with the P-value and T-statistic indicators, which give us information about the statistical significance of factors.
Unfortunately, our sample person will find it difficult to know in advance how their potential partners perform on a large number of essential parameters and will also find large amounts of data difficult to evaluate. However, there are dating apps where users enter some of their characteristics. If our person created their model for choosing a mate, they could choose according to it.
Problem 4: We run into another problem, which is the quality of the data, as potential mates are likely to lie a lot about themselves. We face the same problem with stocks, and so for a functional analysis, we need to get data from good quality sources, which are not cheap.
Using machine learning
We are finally reaching the top of our pyramid. We know how to use individual factors and we know how to build multifactor models. We also hired a programmer who made us a good app, and with great marketing, we got a lot of potential partners into it. We had a detective check them out and we know they are telling the truth about themselves, i.e. the data is good quality. We have been using our multifactor model for some time, but we are still not satisfied with the results.
So we decide to use machine learning. This will help us both discover which factors are most correlated with each other and can dynamically adjust the weights of individual factors so that we choose the action or partner with the greatest potential to make us happy at any given moment.
We also trained the machine learning model not only on us and only on the years 1990-2009, but on several people, from different countries, at different times. Ideally, we train the model on data from medieval Africa, test it on people from Australia, and if it works, start using it ourselves. First just for dating, and then for long-term relationships.
In the first step, we trained a model revealing only linear dependencies. It already made us happier, but we want more, and in the next step we will therefore start with non-linear models describing the changes of variables, which at first glance seem chaotic, and unpredictable, just like human behavior! It can thus better reveal, for example, how one factor affects another or which factor is really important in the given conditions, when this connection is not obvious at first glance to an investment analyst or to us. Or, for example, we don’t have experience with it yet, because people usually only get this experience at a later age.
Conclusion
Human analysts are not able to monitor thousands of factors and their development over time, evaluate and see all connections. Quality software will help them with this. The hypothesis seems to be confirmed by the long-term results of investment companies. Those that use machine learning perform better than those that don’t. Paradoxically, there are not many companies using AI in the area of investments. But, for example, Renaissance Technologies has been successfully using “AI” since 1978. Our Analytical Platform since 2020.
In the relationship world, it’s a bit more complicated. What do you think after reading the article, could the use of machine learning in the real world fulfill the initial hypothesis in the field of love as well? We will be happy if you contribute to us in the comments.
[1] Recall this point in the Using Machine Learning section on training models in different parts of the world.